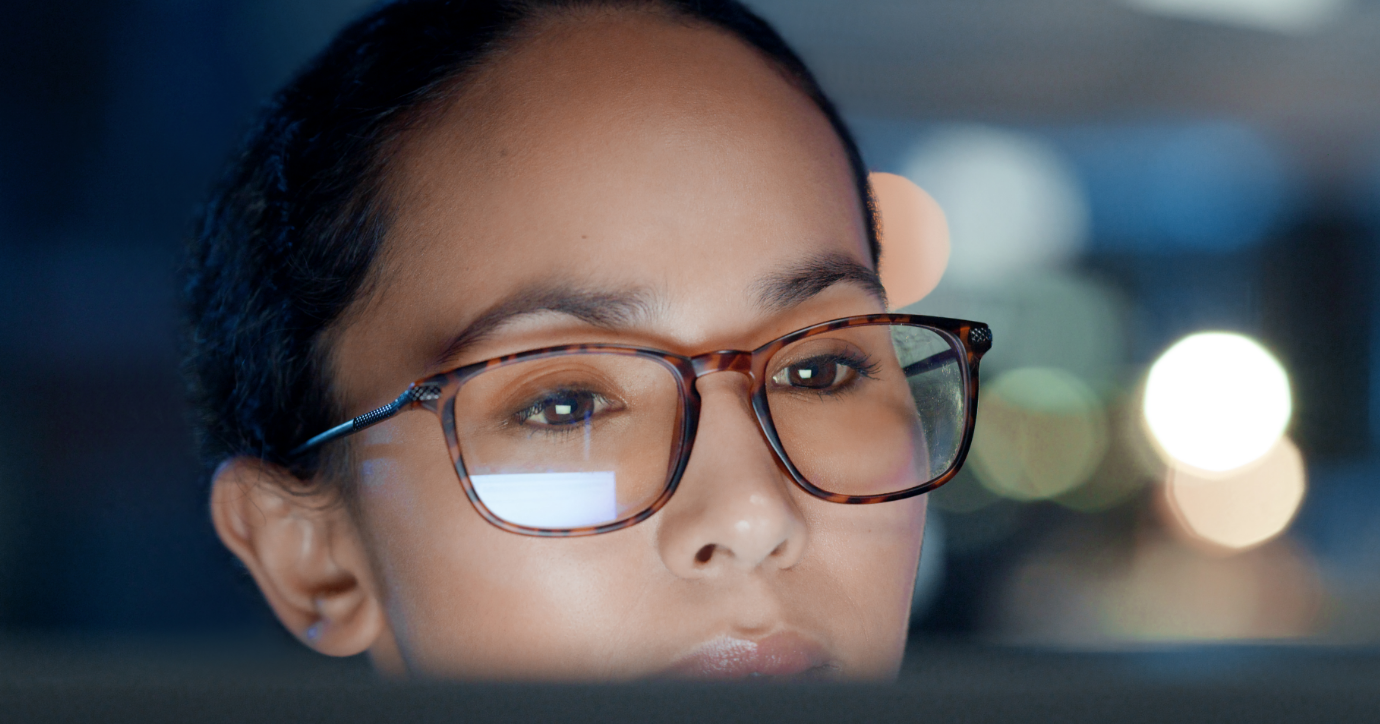
安德鲁•摩根, 6point6的数据主管, believes the data supply chain will make or break any organisation. 在这里, 他强调了采用整体方法进行数据实体匹配的好处,以应对“极其复杂”的数据环境带来的挑战.
通过数据实体匹配增强业务决策
在今天的数字世界, 数据的质量和弹性可以说是做出明智的业务决策和提供卓越服务的最重要的成功因素. 从原始数据中快速准确地推断出有价值的见解,使组织能够应对复杂的挑战,并降低不准确的风险, 潜在的破坏性结果.
优化数据供应链
从原始数据中得出见解的过程需要创建一个数据供应链,包括3个阶段:
- 创建、获取和收集原始数据
- 原始数据的转换和集成, including entity matching which links records together into a holistic and improved data set
- 最终数据产品的消费和分析
开发一个中央数据收集策略是一种当代最佳实践,用于协调团队如何跨组织的流程和事务捕获信息. 这种方法产生结构良好的数据集,支持用于多种目的的快速和准确的分析,并减少诸如重复记录或丢失信息等问题的可能性.
然而,许多组织, 比如中央政府, 是否有来自众多收集系统的大型遗留数据集,每个系统都有自己的细微差别, 质量的挑战, 系统更新生命周期和漂移的使用模式. The data is typically gathered from a complex landscape of computer systems, 每个都是为单一服务设计的. This disparity leads to data discrepancies that are hard to identify, 跟踪, or resolve – adding to the risk of data analysis yielding flawed conclusions such as mistaken identity. 认识到这种情况的惊人复杂性, consider that the UK government has provided public access to nearly 25,000个数据集.
在数据策略中优先考虑个人
Designing services with a user-centred approach prioritises a user’s experience and satisfaction. 例如, 就公共部门服务而言, 许多政府机构奉行“以民为先”的理念,以市民对服务的满意程度作为衡量成功与否的基准. This philosophy provides an opportunity to demonstrate operational efficiency and build reputational excellence.
In the case of digital services, users want a seamless and positive online experience. 提供一个中央公共服务门户,个人可以通过它管理他们当前和历史的数据,如驾驶执照, 护照或医疗服务提供者, is a significant convenience both in terms of access and reusing information for new transactions.
Security and privacy are paramount and data protection laws will apply to processing of personal data. 错误地将数据记录归因于公民或未能将记录与公民联系起来,这充其量是不方便的,并可能产生破坏性后果, 导致用户满意度低,并给参与开发和运营服务的各方带来声誉风险.
These expectations of service necessitate strict data governance and data sharing across departments. 然而, 将无数不同的数据集整合在一起所涉及的复杂性和不同的考虑因素不应被低估. Reliably creating a single view of citizen transactions across public sector services is a critical challenge. 这就是为什么政府部门和机构越来越重视可信的数据专家来开发最佳解决方案.
集成原始数据与数据实体匹配
数据实体匹配是一种强大的方法,您可以在数据供应链的第二阶段使用它来处理不同的原始数据源. 通过这种方法, 您可以查询多个数据存储,以识别最有可能与感兴趣的实体相关的记录——实体是数据科学工具可以作为单个数据单元处理的唯一对象, 比如一个人, 学校, 产品, 发票或化学品.
Methods of data entity matching are evolving as technology progresses. 简单的基于规则的匹配是一种基本方法, 然而,这种方法不能轻易区分真阳性(正确归属于某一实体的记录)和假阳性(错误归属于某一实体的记录)。. 对于大型数据集, the level of uncertainty in the results can rule the sole use of this method out.
Machine learning approaches use contextual information to achieve better accuracy and efficiency. 例如, when trying to entity match records associated with differing names (Janine Smith, 简•史密斯, J. 史密斯)给个人, AI can identify a name’s language of origin and know how it may vary within that language or culture, as well as other identification fields such as dates of birth and addresses.
Machine learning approaches also enable matching names across languages. 例如, 执行安全检查可能需要对个人姓名使用不同语言或文字的记录进行实体匹配. In addition to using text, data entity matching increasingly uses biometric recognition.
Current data matching software offers a tailored approach and high accuracy
The optimal data entity matching approach depends on both the data sources and the project objectives. Developing a tailored and overarching plan that embraces the sources, 数据的整合和未来利用是成功的关键.
自动化复杂的数据匹配任务显然提供了许多好处,例如快速处理大量数据. 然而, human intervention is still required to investigate uncertain matches. 它是实体匹配软件的准确性, which is crucial to reducing the number of records requiring human review.
系统包括 玫瑰Babel Street®将人类知识内置到人工智能(AI)和十多种特定的语言算法(如语音)中, 音译拼写变化, 无序的名字, 和昵称-提供一个快速和智能的匹配系统,从许多不同的角度解释和分析数据. 对于每一个比较, 玫瑰 produces a match confidence score and an explanation of what factors went into the calculation. 这种可解释的人工智能使用户能够显著扩展和微调系统的匹配行为,以反映数据的特定性质, 一个组织的语言变化和业务优先级. 这种灵活性和针对性有助于优化结果的准确性,并最大限度地减少假阳性和假阴性(应该归因于实体但实际上不是的记录)。.
Other types of system focus on implementing specialised algorithms to do probabilistic record linking. 通常这些类型的系统将使用Fellegi-Sunter匹配算法,该算法为用户提供可解释的概率匹配. 司法部已经公开了一个 本系统的实现.
Both systems use score thresholds that indicate confidence in the matches, so users can validate and tune the record matching to a threshold that provides trusted linking of records. 例如, 分数高于84%将被认为是匹配的, 而低于76%的分数则不会, 在验证阶段,一组人类测试人员将调查落在这些阈值之间的结果, to review exactly the cutoff that maximises the records linked but minimises errors in linking.
一旦记录被链接和整合, these systems allow the most highly complex and labour-intensive searches and assessments of names, 地址和日期将自动处理并实时处理. The time-saving benefits from such automation, accuracy and reliability are considerable. 洞察的即时性也有助于更明智的决策,并可以防止不法行为,如欺诈活动.
结论
组织需要高质量的数据供应链,以便在当今的商业环境中有效运作,并准备好应对新的机遇. 数据供应链通常直接决定组织提供和支持的最佳服务标准, 及时的商业决策. They also drive holistic operational efficiencies and mitigate risks which could cascade and impact services.
Data entity matching is a crucial component of a data strategy for all organisations that process large, 不同的数据集. 它通过允许组织从许多不同的来源收集数据来构建和采取更大的行动,从而增强了数据收集策略. 通过将记录有效地连接在一起, organisations are able to better observe a 360 degree view of their customers in the wider world they operate in, offering significant benefits for transforming the way your organisation works.
即使有足够的人员和资金, it’s often harder than expected to implement these data entity matching systems. 技术挑战并不是唯一的困难, as the change approach and smooth transition to new processes can also be difficult to plan and implement. 与一个值得信赖的合作伙伴合作——他知道如何开发整体数据策略和优化数据实体匹配工具——可以实现您的“公民优先”愿景.
十大网博靠谱平台巴别塔街
Babel Street是世界上最先进的身份情报和风险操作值得信赖的技术合作伙伴. Babel Street Insights平台提供先进的人工智能和数据分析解决方案,以缩小风险-信心差距.
欲了解更多信息,请访问 babelstreet.com.
安德鲁•摩根
安德鲁·摩根是6point6的数据总监. 他是《十大网博靠谱平台》一书的作者, 一本学习可扩展数据科学的教科书, 并且还发布了一些开源工具. 他有超过25年的数据项目经验, Andrew now draws on his deeply technical experience to manage and lead high performing data teams.